
Sensitivity Analysis of Albedo Assumptions in Urban-Scale Solar Irradiance Simulations
ⓒ 2025. KIEAE all rights reserved.
Abstract
Urban-scale solar irradiance simulation is complex owing to the influence of surrounding urban morphology. Existing studies have often oversimplified surface albedo by assuming a uniform, generic value, failing to reflect real-world variations. This study quantifies the impact of albedo assumptions on simulation results, focusing on building-integrated photovoltaics (BIPV) on vertical façades—an advancement beyond previous studies centered on rooftop photovoltaics.
Urban-scale solar irradiance simulations were conducted using 10 geometrically identical models with varying surface albedo settings. A synthetic model representing building stocks with realistic albedo parameters was developed as a baseline for comparative analysis. Nine alternative models with different albedo assumptions were generated to assess the sensitivity of annual solar irradiance predictions on exterior façades. Simulations were performed using ClimateStudio. To further evaluate the impact of the surrounding urban context, a novel indicator—Weighted Mean Albedo Difference (WMAD)—was introduced.
Albedo variations from the baseline model had a minimal impact on rooftop solar irradiance, aligning with previous research. However, a significant influence on solar irradiance was observed for vertical façades, directly affecting BIPV energy yield. Sensitivity to albedo assumptions strongly correlated with surface orientation and surrounding albedo distribution. Greater discrepancies between simplified assumptions and realistic values resulted in higher simulation errors. These findings underscore the critical importance of precise albedo representation in urban-scale models to ensure reliable BIPV potential estimations.
Keywords:
Urban Building Energy Modeling (UBEM), Building-Integrated Photovoltaics (BIPVs), Albedo, Sensitivity Analysis1. Introduction
1.1. Research Background and Purpose
According to a report by the International Energy Agency (IEA) in 2019, the building sector accounts for 28% of global energy-related CO2 emissions, and direct emissions from the building sector are expected to decrease by 75% in the 2050 carbon neutrality target scenario. This indicates that carbon reduction in the building sector is absolutely required for achieving carbon neutrality [1]. In line with, the Ministry of Land, Infrastructure and Transport of South Korea established the “2050 Carbon Neutral Roadmap” in 2021 and presented the dissemination of zero energy buildings (ZEB) as one of its key policy tasks. Accordingly, ZEB has become mandatory in stages since 2020, and ZEB grade 5 certification will also be required for private-sector buildings with a total floor area of 1,000m2 or larger from 2025 [2]. To realize ZEB, the essential requirement is to improve the energy efficiency of buildings and secure energy self-sufficiency through renewable energy power generation within the site (at least 20%). Photovoltaic (PV) systems, which have consistently improved power-generation efficiency and reduced costs as urban renewable energy sources, have attracted attention as the most practical solution for energy self-sufficiency. Representative PV systems are rooftop PV systems, which minimize the shades caused by surrounding buildings and geographical features, maximizing the total solar irradiance that reaches PV panels. For high-rise buildings (both commercial and residential buildings) that are densely located in urban areas and contribute significantly to carbon emissions, however, achieving energy self-sufficiency using rooftop photovoltaics alone is difficult because the rooftop area is limited compared to that required for high energy consumption. This tendency intensifies for buildings with a high total floor area ratio compared to the rooftop area available for installing rooftop photovoltaics. Therefore, building-integrated photovoltaics (BIPV), which can secure structural stability while maximizing the use of building vertical façades, have attracted attention as a more practical alternative [3].
To assess the potential of BIPV, various studies have focused on BIPV power-generation simulations over the past 10 years [4~7]. Previous studies, however, have a common limitation in that they have applied simplified assumptions for the surface solar reflectivity (albedo) within urban models. Most studies have constructed urban energy models by uniformly setting urban surface albedo to 20% without sufficiently considering different building materials, urban forms, and environmental conditions. This may be because obtaining measurement-based albedo data is practically difficult, and no systematic database on albedo for building exterior materials is available. Nevertheless, previous studies have reported cases with no significant error between the predicted and measured values of photovoltaic potential and solar irradiance, despite the application of simplified models [8]. This may be because most studies have focused on solar irradiance on horizontal and inclined rooftop surfaces where the slope angle is limited to 0~30 degrees, and the influence of surrounding structures is not dominant. Additionally, the possibility that the results were caused by model parameter correction cannot be ruled out. In the case of BIPV and building-attached photovoltaics (BAPV) that are installed on vertical and inclined surfaces outside buildings, however, the complex albedo distribution of surrounding structures makes predicting solar irradiance and solar power generation through simulation more complicated.
Against this backdrop, this study aims to conduct a quantitative and systematic analysis of the behavior of predicted solar irradiance on the building external surface according to urban building energy modeling (UBEM) albedo settings to establish more practical strategies for improving the energy self-sufficiency of urban buildings.
1.2. Research Methods and Procedure
In this study, sensitivity analysis was conducted based on the errors between the baseline model and alternative models to analyze the effects of building orientation, surrounding buildings, and albedo variations on solar irradiance in the urban environment (see Fig. 1.). The baseline model was constructed based on the building data extracted from the digital twin city model of S-Map (a 3D platform that integrates Seoul spatial information) [15], while the alternative models were generated by adjusting the albedo value within the range of 0.1~0.9. The urban model was constructed at the Level of Detail 1 (LoD1) using the geographic information system (GIS) data of the seamless digital map from the National Geographic Information Institute (NGII). Solar irradiance was simulated using Climate Studio’s Radiation Map plug-in tool, and annual solar irradiance was calculated by placing a sensor grid at 2-m intervals on the five external surfaces (east, west, south, north, and rooftop) of 20 target buildings. The annual solar irradiance data were then post-processed using Python and ArcGIS Pro, and Weighted Mean Albedo Difference (WMAD)-solar irradiance error correlation analysis was conducted to quantitatively evaluate the impact of the albedo of surrounding buildings on the target building. Based on the analysis, an attempt was made to quantify the impact of albedo settings on simulation results and the effect of simple assumptions for the building context on the uncertainty of the results.
2. Theoretical Consideration
2.1. Consideration of Albedo in Previous Studies
In most previous studies, the urban surface albedo was set in the range of 0.2~0.35 rather than using measured values, and the most conservative value of 0.2 was frequently used (see Table 1.). While researchers were aware that the albedo value may vary depending on the surrounding environment and conditions, they applied simply corrected values with no clear evidence based on the reason that simplification of calculations, bias minimization due to estimation, or the albedo value has no significant impact on the results. However, using a fixed value of 0.2 is not appropriate because the albedo value may increase to 0.2 in temperate regions, 0.5 in arid regions, and up to 0.9 in snowy regions depending on local characteristics [13]. Moreover, solar energy may increase by up to 37% by considering albedo by terrain. Additionally, reflected radiation may have a significant impact for high albedo values of 0.6~0.7 or slopes, and using a fixed albedo value may degrade the reliability of the results [14].
3. Simulation Modeling and Analysis Method
3.1. Case Study Site Selection and Modeling
In this study, the baseline model and alternative models were constructed based on the process in Fig. 2. using the GIS data of the seamless digital map provided by NGII [16]. The purpose of this study was to quantify the impact of the urban surface albedo setting error on the simulation solar irradiance prediction error, rather than the prediction accuracy of the solar irradiance simulation model itself; thus, the baseline model was constructed with more attention to plausibiility than accuracy. A building group in a scale of a complex was selected as a study site, and geometric information and albedo values were estimated through general building characteristics and observations because accurately estimating or measuring the geometric models and albedo of urban-scale buildings and terrain is difficult. For the alternative models, the predicted solar irradiance error compared to the baseline model was quantified by applying the same albedo value to the entire urban surface, as in previous studies.
In this study, a residential area in Seoul with large altitude differences in terrain and clear differences in albedo between buildings was selected as a case study site (see Fig. 3.). Additionally, only buildings with a total floor area of 1,000m2 or larger, which are the targets of mandatory ZEB certification, were selected as research targets. This is because the buildings were highly likely to be equipped with BIPV as securing a certain energy self-sufficiency rate under the ZEB roadmap was necessary and because they were determined to be the first research targets in realizing carbon-neutral architecture owing to high total energy consumption. Finally, 20 representative buildings among the selected buildings were analyzed (see Fig. 4.).
Building modeling was performed based on building area polygons and floor information using ArcGIS Pro. Since no information was provided on the buildings’ floor heights, a typical floor height of 3 m was assumed. It was multiplied by the number of floors, and the building’s polygon geometry was protruded to generate LoD1-level 3D geometry. A 3D model that can be used in solar irradiance simulation was constructed.
To reflect urban terrain, a terrain model was constructed based on the contour information of the seamless digital map using ArcGIS Pro. A triangulated irregular network (TIN) was formed using point data that included the altitude information of contour lines. It was converted into a digital elevation model (DEM), a pixel-based model. Finally, a realistic model was constructed by protruding the formed road polygons at a height of 0.01 m on the generated terrain model.
Albedo information was extracted using S-Map’s building image data. Based on this, a realistic baseline model was generated. Each albedo value was set based on the material information of Spectral DB [17]. It was applied to concrete (29.82%), brick (13.84%), white paint (69.68%), and curtain wall (8.30%) buildings. A single value was assigned to terrain (7.38%) and roads (11.39%) (see Table 2. and Fig. 5.).
Albedo scenarios (0.1~0.9) were set to cover a range from red bricks (albedo: 0.104), a representative low-albedo exterior material, to white exterior wall plaster (albedo: 0.866), a high-albedo exterior material. They were applied to buildings, terrain, and roads, with a focus on the quantitative analysis of errors that occur in the broad albedo range.
Emphasis was given to a comparison with albedo 0.2 among the set scenarios to closely evaluate the difference from the albedo value commonly applied in previous studies [17,18]. This evaluation is critical for examining the reliability of simulation results. Each scenario applied the material properties specified in Table 3., and the same material properties except for albedo were applied.
3.2. Solar Irradiance Simulation
In this study, the annual solar irradiance of building façades was quantitatively evaluated using the Radiation Map tool of ClimateStudio, a Rhino plug-in. During the simulation process, a sensor grid was placed at regular intervals to accurately calculate annual, monthly, and hourly solar irradiance that reaches building façades and rooftops. Based on the evaluation, the spatiotemporal fluctuations of solar irradiance by surface were analyzed, and design was performed to evaluate the solar potential of the building envelope more systematically.
ClimateStudio is a tool that performs physically accurate solar radiation simulations based on the path-tracing algorithm. This algorithm probabilistically samples ray and traces the path of light, making accurately modeling multiple reflection and irregular reflection effects, as well as direct solar radiation, possible [19]. These characteristics are favorable for realistically reflecting the reflected light and indirect illuminance effects caused by complex building layout in urban environments.
For an accurate analysis, the initial input values of simulation were systematically set. Key input values include climate conditions, building material information, the sensor placement method, and the simulation period (see Fig. 6.).
- ∙ Climate data: The solar and sky irradiance of Shinyongsan TMYx(2004~2018) weather data was reflected for simulation.
- ∙ Sensor placement: Sensors were placed at 0.2-m intervals on rooftops and façades.
- ∙ Simulation period: Annual, monthly, and hourly solar irradiance was calculated.
3.3. Analysis Method
In this study, the building envelope was classified into five façades (east, west, south, north, and rooftop) based on its normal vector N=(Nx, Ny, Nz).
First, it was classified as east and west façades for |Nx| ≥ |Ny|; later, it was classified as south and north façades . The east and west façades were distinguished as east façade for Nx ≥ 0 and west façade for Nx < 0. The south and north façades were distinguished as north façade for Ny ≥ 0 and south façade for Ny < 0. Finally, the façades were classified as rooftop if the Nz value was positive (see Table 4. and Fig. 7.).
In this study, a new indicator was proposed to quantitatively analyze the effects of the target building’s façade orientations and the surrounding buildings’ albedo on the solar radiation environment of the target building. A semicircular area with a radius (R) of 100 m for a specific direction was set around the target building (see Fig. 8.), and the façade area (Ai) weighted mean of the difference between the baseline model albedo (αi) and alternative model albedo (α0.2) was calculated for all buildings included in the semicircular area using Eq. 1. The indicator was calculated for 20 buildings by façade direction, and a comparative analysis with the albedo difference was conducted.
(Eq. 1) |
- where Ai: façade area (m2)
- αi: individual building albedo in the baseline model
- α0.2: alternative model albedo
- R: radius (m)
In the case of the building in Fig. 8., the semicircular area was set in the southern direction, and WMAD was calculated to analyze the building’s south façade. In the calculation process, building 4 had a larger impact on the results of the target building than on those of other buildings owing to its façade area (A4) of 1,000m2. This is the result of calculating the effects of surrounding buildings on the target building based on the façade area (Ai) (see Eq. 2).
(Eq. 2) |
4. Sensitivity Analysis
4.1. Sensitivity Analysis for Alternative Models with Albedo values from 0.1 to 0.9
The error with the baseline model was analyzed by building façade, while the albedo value increased by 0.1 from 0.1 to 0.9. As shown in Fig. 9., the error showed a tendency to exponentially increase from negative to positive values as the albedo value increased. In particular, when the albedo value was 0.9, the maximum error of annual solar irradiance reached 505kWh/m2·yr on the west façade.
However, the rooftop showed relatively insignificant average and maximum errors (3.11 and 7kWh/m2·yr, respectively). This may be because the analyzed target buildings were less affected by the surrounding environment as they were relatively higher than the surroundings. In general, higher buildings are less affected by the reflected light from surrounding buildings. Thus, low sensitivity to albedo changes can be interpreted as a natural outcome. Conversely, different results may occur for relatively low buildings that are significantly affected by surrounding buildings. Therefore, caution should be exercised when the results of this study are generalized for all building types.
4.2. Error Analysis between Baseline and Alternative Models under the Application of Albedo 0.2
This study attempted to examine the validity of albedo 0.2, which had been conventionally applied in previous studies. To verify this, a sensitivity analysis was conducted based on albedo 0.2.
The sensitivity of each building façade was evaluated by analyzing the errors between the baseline and alternative models under the application of albedo 0.2. According to the graph presented in Fig. 10., the median values of the absolute error were 63.20, 68.31, 66.86, 67.33, and 4.70kWh/m2·yr for the east, west, south, and north façades and the rooftop, respectively. While the east, west, south, and north façades exhibited similar error ranges, the rooftop showed a relatively low error.
The effects of the characteristics of certain buildings on sensitivity were examined through the analysis of individual buildings rather than the entire building average. As seen from Fig. 11., the error values of individual buildings were significantly different and did not follow a consistent tendency.
Buildings 1~5, however, showed negative error values, while most of the other buildings exhibited positive error values. The analysis of Fig. 2. and Fig. 5. revealed that the surrounding building albedo of buildings 1~5 was lower than that of buildings 6~20.
Clear differences in sensitivity by façade were also observed even within the same building. For example, the error of the west façade of building 10 was as high as 218.25kWh/m2·yr, while the error of the south façade was only 31.92kWh/m2·yr.
The tendency of sensitivity could be partially confirmed in the above results, but its generalization has limitations. The WMAD indicator was used to evaluate the sensitivity tendency by reflecting the characteristics of surrounding geographical features. Additionally, outliers with an absolute value of 2.0 or higher were removed using Studentized Residuals to further examine the general tendency between WMAD and the solar irradiance error.
In the analysis results, the coefficient of determination (R2) was 0.5102, 0.8543, 0.7887, and 0.6128 for the east, west, south, and north façades, as shown in Fig. 12. Since the coefficient of determination of the east façade was relatively low (0.5102), clearly verifying the impact of the albedo of surrounding buildings on the simulation error was difficult, but a certain tendency could be identified. Moreover, we estimate that the data that deviated significantly from the trend line could be more significantly affected by topographic factors or shading than by surrounding buildings.
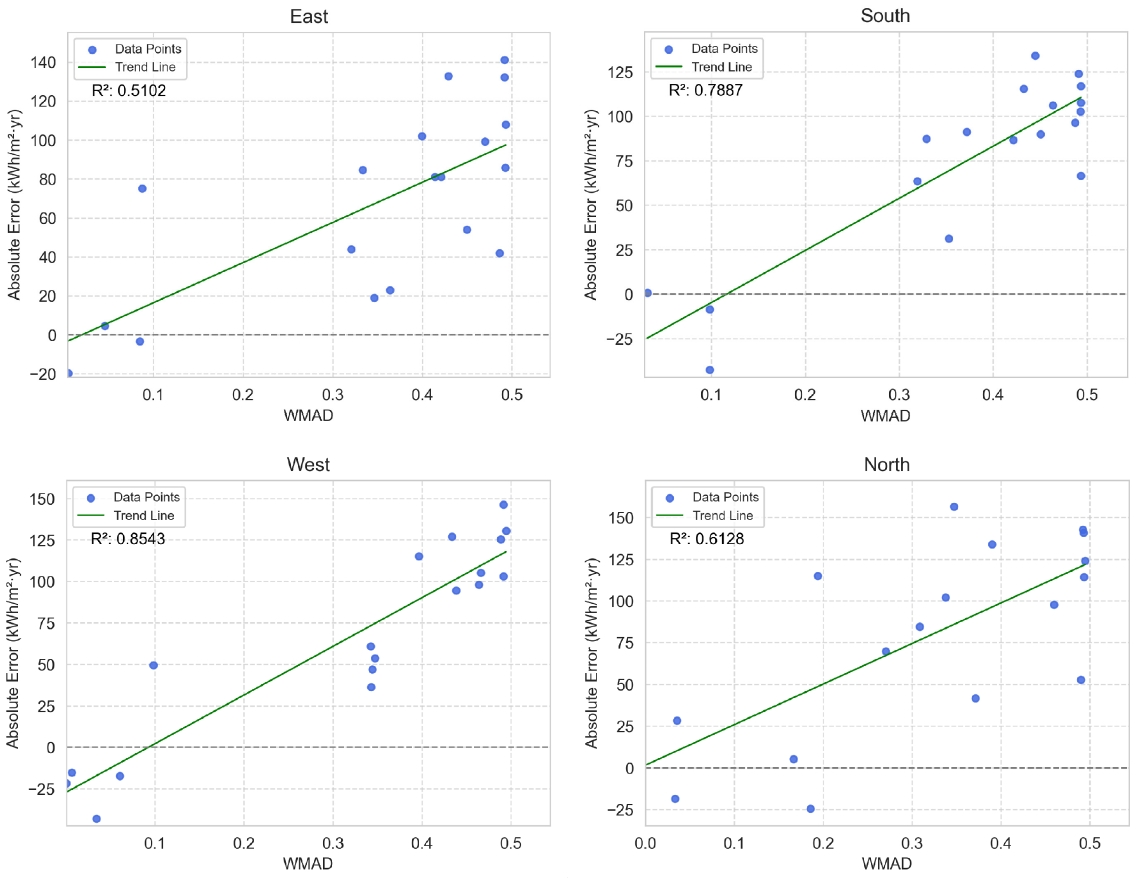
Correlation analysis of WMAD and solar irradiance prediction error for façade orientations (E, W, S, N)
For example, building 5 showed an error of -46.46kWh/m2·yr when WMAD was 27.63, and Fig. 5. shows that the building was affected by the inclined terrain. Thus, the error appears to have been underestimated. In the same manner, building 11 showed an error of -21.99 kWh/m2·yr when WMAD was 48.92, and Fig. 5. confirmed that the building was affected by the inclined terrain in the east. This indicates that topographic factors, such as inclined terrain, as well as the albedo of surrounding buildings may have substantial impacts on the sensitivity of the results of individual buildings [14].
Consequently, the linear relationship by which the albedo of surrounding buildings has a significant impact on the simulation results of the target building could be verified through the WMAD-solar irradiance error correlation analysis. This study also confirmed that changes in the albedo of surrounding buildings may cause substantial differences in simulation values by building.
5. Conclusion
In this study, research was conducted by constructing a baseline model and alternative models to analyze the impact of albedo settings on the sensitivity of results in BIPV simulation. Albedo was adjusted by 0.1 from 0.1 to 0.9 for the alternative models, while the baseline model applied more precise albedo values by reflecting building envelope data based on the S-Map data. In particular, comparative research was conducted to analyze the impact of an albedo value of 0.2, which has frequently been used in previous studies, on the simulation results. Furthermore, the linear relationship between the albedo error distribution of surrounding structures by façade orientation and the predicted solar irradiance error was confirmed by applying Weighted Mean Albedo Difference (WMAD)-solar irradiance error correlation analysis.
In the analysis results, based on the entire building average, the east, west, south, and north façades exhibited similar levels of error sensitivity, but the rooftop showed particularly low sensitivity. This indicates that vertical façades are significantly affected by albedo changes compared to the rooftop in BIPV simulation. Additionally, the analysis of individual buildings and façade orientations revealed differences in error sensitivity depending on the building and showed that a maximum difference of 186.33kWh/m2·yr occurred depending on the façade orientation even in the same building. This provides important implications for façade BIPV design during building construction and remodeling. The BIPV potential of a certain building can be underestimated or overestimated depending on albedo settings for surrounding structures, and it may vary significantly depending on the geometric relationship between the vertical façades of the building and the geographic features facing them. In other words, the energy self-sufficiency rate that can be realized at the same cost may vary depending on the geometrical and optical context surrounding the building. This provides important implications at this point in time, before the substantial expansion of target buildings for ZEB certification in the ZEB roadmap. In a situation where the context surrounding the building significantly affects the cost required to secure energy self-sufficiency, discussions on whether applying a uniform energy self-sufficiency standard by building group is appropriate are required.
Through the WMAD-solar irradiance error analysis, the coefficient of determination (R2) was found to be 0.8543, 0.7887, and 0.6128 for the west, south, and north façades. This indicates that more than 60% of the impact of the albedo of surrounding buildings on the target building’s BIPV simulation results can be explained. In particular, up to 85% of the linear relationship of error sensitivity through WMAD on the west façade could be explained. However, the coefficient of determination of the east façade was relatively low (0.5102); this made fully explaining the simulation error with the albedo of surrounding buildings alone difficult. This may be because the geometrical and optical context of surrounding buildings inherently has nonlinear characteristics. To address this problem, in the future, the nonlinear influence of the surrounding environment must be systematically analyzed by reflecting more sophisticated analytical techniques and additional variables. Moreover, a baseline model was arbitrarily generated based on the images of S-Map, but follow-up research is required to reflect more realistic albedo values. Furthermore, the model’s reliability must be improved by conducting research to compare and correct measured data and simulation results. This will make further improving the reliability of BIPV simulation and more accurately evaluating BIPV performance in real-world environments possible. Finally, the baseline model of 20 buildings set in this study was insufficient in parameters and spectrum to generalize real urban environments with various geometrical and optical characteristics. To address this issue, our future research will (1) expand the scope of research to a wide range of urban areas that include various building types; (2) include the morphological context of urban environments and buildings in research; and (3) propose a multi-parameter model through an index that quantifies the relevant information more accurately than WMAD to secure the scalability of research results.
Acknowledgments
This work was supported by the Korea Agency for Infrastructure Technology Advancement funded by the Ministry of Land, Infrastructure and Transport in 2024 (No.RS-2024-004099 53).
References
- IEA (International Energy Agency), Perspectives for the clean energy transition, 2019.
- Ministry of Land, Infrastructure and Transport, National land and transport 2050 carbon neutrality roadmap, 2021.
-
U. Perwez et al., Multi-scale UBEM-BIPV coupled approach for the assessment of carbon neutrality of commercial building stock, Energy & Buildings, 291, 2023, 113086.
[https://doi.org/10.1016/j.enbuild.2023.113086]
-
S. Anselmo, M. Ferrara, Trends and evolution of the GIS-based photovoltaic potential calculation, Energies, 16, 2023, 7760.
[https://doi.org/10.3390/en16237760]
-
P. Florio et al., Designing and assessing solar energy neighborhoods from visual impact, Sustainable Cities and Society, 71, 2021, 102959.
[https://doi.org/10.1016/j.scs.2021.102959]
-
Y. An et al., Solar energy potential using GIS-based urban residential environmental data: A case study of Shenzhen, China, Sustainable Cities and Society, 93, 2023, 104547.
[https://doi.org/10.1016/j.scs.2023.104547]
-
Z. Liu et al., Integrated physical approach to assessing urban-scale building photovoltaic potential at high spatiotemporal resolution, Journal of Cleaner Production, 388, 2023, 135979.
[https://doi.org/10.1016/j.jclepro.2023.135979]
-
H.G. Beyer et al., Assessment of the method used to construct clearness index maps for the new European Solar Radiation Atlas (ESRA), Solar Energy, 61(6), 1997, pp.389-397.
[https://doi.org/10.1016/S0038-092X(97)00084-4]
-
J.A. Jakubiec, C.F. Reinhart, A method of predicting city-wide electricity gains from photovoltaic panels based on LiDAR and GIS data combined with hourly Daysim simulations, Solar Energy, 93, 2013, pp.127-143.
[https://doi.org/10.1016/j.solener.2013.03.022]
-
H.T. Nguyen, J.M. Pearce, Estimating potential photovoltaic yield with r.sun and the open Source geographical resources analysis support system, Solar Energy, 84, 2010, pp.831-843.
[https://doi.org/10.1016/j.solener.2010.02.009]
-
K.T.N Ihsan et al., City-level solar photovoltaic potential using integrated surface models and himawari satellite in Japan, Renewable Energy, 319, 2024, 114552.
[https://doi.org/10.1016/j.enbuild.2024.114552]
-
J. Polo et al., Photovoltaic generation on vertical façades in urban context from open satellite-derived solar resource data, Solar Energy, 224, 2021, pp.1396-1405.
[https://doi.org/10.1016/j.solener.2021.07.011]
- Y. Kotak et al., Impact of ground albedo on the performance of PV systems and its economic analysis, The 7th International Conference on Solar Radiation and Daylight, Celje, Slovenia, 2015.
-
J.A. Duffie, W.A. Beckman, Solar engineering of thermal processes, 4th ed., Hoboken, NJ: John Wiley & Sons, 2013.
[https://doi.org/10.1002/9781118671603]
- Seoul Special Metropolitan City, S-MAP, https://smap.seoul.go.kr/, , 2025.
- National Geographic Information Institute, NGII map service, https://map.ngii.go.kr/mn/mainPage.do, , 2025.
- Spectral Materials Database, https://spectraldb.com, , 2025.
-
J.A. Jakubiec, Data-driven selection of typical opaque material reflectances for lighting simulation, LEUKOS, 19(2), 2023, pp.176-189.
[https://doi.org/10.1080/15502724.2022.2100788]
- Solemma, ClimateStudio, https://www.solemma.com/climatestudio, , 2025.