
Archetype Modeling Uncertainty and Bayesian Calibration for Campus-scale Analysis of Seoul National University Buildings Energy Use
ⓒ 2022. KIEAE all rights reserved.
Abstract
Considering the gap in research on uncertainties in urban building energy modeling, with specific regard to archetype building development, this study seeks to first throw light on archetype modeling’ sources of uncertainty, and second, solve these issues with the Bayesian calibration method.
By using a case area of Seoul National University, this paper models five 3D archetype buildings and estimates their monthly energy use intensities on EnergyPlus. The simulation results are modified with the Bayesian calibration technique, compared against existing, observational data before and after the Bayesian calibration, and further validated by computing the coefficient of the variation of the root mean square error.
Findings show that the Bayesian calibration may be considered to address uncertainty as it improves the simulation results; however, it is not a one-size-fits-all solution to uncertainties, caused by the lack of some parameters, not added to the model, which are crucial to the archetype characterization. Overall, this paper contributes to the research on urban building energy modeling-related uncertainties, hoping for urban planners to consider sources of uncertainty during the archetype characterization stage and make the adequate modeling decisions in the name of sustainable urban growth.
Keywords:
Building Energy Modeling, Building Archetype, Uncertainty, Bayesian Theory키워드:
빌딩 에너지 모델링, 건축물 유형, 불확실성, 베이지안 이론1. Introduction
Increasing urban growth calls for actions in sustainable urban planning. Decision making has focused its efforts at the municipal and neighborhood levels overshadowing the contribution of buildings in energy use[1]. Refining urban strategies, that highlight building stock energy supply and demand, is crucial to provide recommendations for sustainable urban growth. This has become possible with the development and practice of bottom-up urban building energy modeling (UBEM), which supports the measurement of buildings’ energy supply and demand for various spatiotemporal resolutions; and archetype modeling, the method of interest in this study[2]. This technique models building energy consumption for one or more main buildings by selecting and incorporating some specific parameters, which impact the building energy stock. The modeling outcomes for this small range of representative buildings can be scaled up to typify a larger building stock of wider spatial scopes that meet the designated archetypes’ descriptions[3]. However, using the values of one building to generalize and estimate the dwelling stock of a larger area represents a delicate task. One single issue with the input and specification of the archetype model parameters can disturb modeling and lead to wrong simulation results, which are further extrapolated to a larger group of buildings. Uncertainty, which may accordingly arise, has been challenged by employing probabilistic methods, including the Bayesian calibration technique[4-6].
Since UBEM studies commonly fail to discuss uncertainties and tend to highlight one kind of uncertainty, this research sought to: (i) throw lights on archetype modeling’ sources of uncertainties, and (ii) solve those issues with the Bayesian calibration method[7]. Hence, by using Seoul National University (SNU) campus as case area, this study asks the following research question:
How and to what extent can uncertainty be addressed by the Bayesian calibration for campus energy analysis?
The paper employs hypothesis testing where the null hypothesis is:
H0: is the simulation results’ CVRMSE (coefficient of the variation of the root mean square error) close to the ASHRAE (American Society of Heating, Refrigerating and Air-Conditioning Engineers) guideline’s recommended value of 15%?
The CVRMSE represents an appropriate results’ validation indicator that will measure the effect of the Bayesian calibration on the changes in the simulation findings, pre-and-post calibration[8]. In line with the research question, this paper first explores the UBEM literature regarding the use of bottom-up approaches, archetype method and Bayesian calibration, as well as uncertainty. Second, authors will introduce the methodology and third, provide a case study of SNU, with 3D models of five archetype buildings and their energy use intensities, as a result of running simulations on EnergyPlus, which are further modified with the Bayesian calibration, compared against observational data and validated. Last, the paper discusses the findings’ academic implications and suggests research improvements.
2. Literature review
2.1. Urban building energy modeling
Urban Building Energy Modeling (UBEM) aims at first, quantifying the energy contribution of buildings in urban settings for various spatiotemporal resolutions and second, providing urban planning and support, and thermal comfort enhancement[2].
According to Johari et al., the UBEM workflow, like shown on Fig.1., consists of several phases: (i) the model development, (ii) the model simulation, (iii) validation and calibration, and (v) data visualization. Model development integrates 3D city modeling with archetype development by combining the buildings geometric characteristics and their surroundings (i.e. floor and rooftop areas, volumes, façade) with non-geometrical attributes (i.e. materials, HVAC system, occupancy), which determines the energy building stock[9]. These new inputs are exported to the simulation engine integrating climatic conditions. Through the core engine, the thermal models, zoning and further simulation are run, generating the energy demand, which can be further, and should be, validated with existing findings, calibrated to different temporal scales, and visualized[9-10].
Swan and Ugursal have put forward two categories of methods for UBEM—the top-down approaches and the bottom-up approaches. On one hand, the former investigates the building area as an energy sink and enacts variables that influence usage in order to forecast changes. On the other hand, the bottom-up approaches identify energy demand contributions of consumers by relying on statistical and engineering techniques[3]. The current understanding of energy flows is conducted in a top-down fashion. While these models may be appreciated for offering energy forecasting of one area, if one building was subject to be replicated and/or converted on a larger scale; they extrapolate from the existing environment which results in a reduced suitability in the advent of more integrated scenarios or peculiar areas[1]. Bottom-up approaches offer a metal-scale planning solution to the disadvantages of top-down models by implementing physical models of thermal mass flows of buildings and their surroundings to estimate energy use for a set of buildings[1].
2.2. Bottom-up approach
The bottom-up approach emerged with the objective to ‘identify the contribution of each end-use towards the aggregate energy consumption value of the residential stock’[3]. This approach is divided into two branches contingent on the evaluation method of energy use: statistical tools and engineering techniques. Statistical methods focus on energy use values from a sample of buildings and regresses end-uses with energy consumption while engineering techniques use macroeconomic and energy indicators of the building and end-uses’ attributes in order to compute energy consumption[3].
Engineering techniques are commonly divided into three sub-techniques: (i) distributions, (ii) archetypes and (iii) samples. The first one estimates each end-use energy consumption by employing appliance ownership’s distribution and use, alongside appliance ratings, which can further be aggregated into residential energy consumption at different spatial levels. The archetype technique incorporates input parameters of buildings (i.e. size, types, construction years) into the energy model after classifying the housing stock in accordance with these parameters. The energy consumption output of building archetypes can then be augmented to constitute the regional and/or national housing stock. Lastly, the sample technique rests upon the input of a sample building data into the model, in which the consumption estimate can also be scaled up to capture an area’s housing stock[3].
Engineering techniques provide advantages in modeling new technologies, learning about end-use energy consumption by appliance types and ratings, and estimating energy trends. Nonetheless, engineering approaches suffer from the assumption of occupant behavior, the necessity for detailed input parameters and computational magnitude[3].
The use of UBEM usually requires software users to manually enter geometric and construction building data, which represents a time consuming and expensive procedure, even more so in the case of a large group of buildings[1]. UBEMs’ consequential reliance on information, especially historical consumption information for bottom-up statistical methods and sophisticated assessment for engineering techniques, has been challenged by the introduction of two methods: (i) the extraction of a sample of buildings and (ii) the formation of archetype buildings[3][11-12].
Nevertheless, archetype development may fall short in validating the estimated predictions against existing results. Archetype building modeling is likely to lead to significant deviation in data findings caused by the difficulty to use a single building that is well enough representing a group of buildings, that especially involve multiple historical, statistics and construction accounts[12-13]. Although archetype characterization may be sophisticated, when based on stochastic values—sole values applied the same to a specific input and employed for each building—they may be unable to inform energy use intensity (EUI) for the buildings pertaining from the same archetype. Data provenance also affects the extent of reliability on the inputs required for the archetype characterization [12][14]. When modeling outputs are different from the actual monitored data, the software user must modify inputs and parameters through several attempts, until the results conform to the existing data. However, this exercise relies on the adjustment of a plethora of variables which can hinder the simulation’s credibility, resulting in uncertainties. Credibility may also be shaken by a lack of appropriate specification of the model inputs—climatic data, parameter sets, information on the control algorithms—which calls for judgement of the values of certain parameters and their calibrations[6].
Uncertainties, which referred to the ‘means of discrete, uniform, triangular or normal probability distributions depending on the input parameters,’ may also be known as errors in urban modeling research and stem from the: (i) incorrect input of parameters, that could be due to the software user’s inexperience or neglect, and incorrect specification of building materials and system parameters; (ii) incorrect model speculations and simplifications, (iii) weak robustness check and numerical algorithms; and (iv) error while inputting the simulation code[4][6]. Oberkampf et al. puts a special emphasis on differentiating uncertainties from errors and claims, that uncertainties may be either aleatory—derived from ‘an inherent variation associated with the physical system or the environment under consideration’—or epistemic, where uncertainty arises from the possibly (non-)existing absence of accuracy resulting from a lack of knowledge[15].
2.3. UBEM hybrid models
Uncertainty analysis (UA) might apply probability distributions to the uncertain parameters during the building design process—architectural design inputs, overheating risk during summer, HVAC systems—attributing a degree of probability in order to estimate unknown or uncertain data[4]. Depending on data availability, probabilistic uncertainty methods rely on robust probability theory and show superiority in either first, offering more accurate and efficient outcomes by changing the existing computational system code, or second, in sustaining a largely-approved model code at a large computational price[16]. Bayesian analysis, known as a ‘statistical inference method in which unknown variables [...] are treated like random variables’, has been used in building energy simulations to address uncertainty and enhance calibration[5][16-19]. The Bayesian analysis seeks to estimate a posterior probability distribution for the results of the regressor, by assigning a probability prior distribution function to the uncertain values. This provides revised distribution functions, achieving posterior distributions that capture a new state of affairs contingent on the experimental data parameters. This method then offers a comprehensive description of uncertainty, for a more solid prediction and stochastic optimization[20].
Using Bayesian theory, Zhao et al. adjusted the data of energy use intensity of the archetype building simulation of a university campus in Liaoning Province, China, with monitoring energy consumption data[13]. This Bayesian approach enhanced both predictions based on the archetype modeling by integrating real consumption information and prediction accuracy for campus building energy consumption and decreasing prediction errors by ten percent.
Sokol et al. also employed the posterior distribution of the yearly calibration, rather than the prior distribution, improved with a Bayesian calibration for four residential buildings in Cambridge, Massachusetts, which identified the missing parameters of annual energy consumption and captured uncertainties while enhancing accuracy of the area’s archetype with a 27 percent point-decrease in error in the EUI distribution mean[14].
The same approach was pursued in a study for a group of test cells in Spain, which decreased differences between originally-monitored and simulation findings of average hourly temperature measurement by approximately 80%, refining model accuracy pre-and-post calibration. Data deviation caused by archetype modeling was addressed by the use of the Bayesian calibration[21].
3. Methodology
3.1. Structure of the modeling system
Fig. 2. presents the structure and data inputs of the modeling system, which unfolds in four main stages: (i) the data preparation model, (ii) the pre-simulation model, (iii) the simulation model, and (iv) the Bayesian calibration and validation model. In the first stage, data originated from the 2D drawing maps, requested from SNU Infrastructure Management Department, which included geometric information of the archetype buildings[22]. The pre-simulation model exported the former data and applied supplementary ASHRAE templates available on EnergyPlus for the occupancy, shading and microclimate effects, resulting in the 3D models of each archetype building. The simulation model generated the archetypes’ monthly, and hourly, energy use intensities (EUIs). The last model proceeded to the Bayesian calibration of the simulation outputs to acquire modified EUIs, which were further compared with the SNU ISD monitored data[23]. The CVRMSE was computed to assess the validity and impact of the Bayesian calibration.
3.2. Building classification
The archetype method downsizes the number of buildings of an area to a sample of fewer buildings that depicts topologically-alike buildings, which allows to ignore certain building parameters which are not available for all buildings and lessen modeling time. Building classification—the first step of archetype modeling which classified buildings by use, construction year and geometric characteristics—is instrumental to energy modeling as energy use alternates according to topology, construction year and so on[24-25]. Land use legislation may be considered to classify dwelling types. Alves et al. employed land use laws and municipal building codes of Belo Horizonte, Brazil, to suggest a building topology classification[26]. Moreover, in evaluating campus energy consumption, scholars may classify buildings by usage purpose[13][27]. Seoul National University Institute for Sustainable Development (ISD) classifies campus buildings by their purposes to model energy use and publish annual reports. As shown on Fig. 3., buildings were classified into nine sub-categories based on SNU ISD’s reports.
In South Korea, insulation laws have been adopted since 1979 and represent an important influencer to building construction[28]. Considering that SNU campus buildings were built in different time periods under different building laws, wall thickness and insulation standards were chosen as classification parameters. This classification method additionally divided buildings into three groups as showed on Fig. 3. Following the two-step classification, SNU buildings were categorized into a total of 26 building archetypes and mapped out with color codes on QGIS (ver. 3.16), shown on Fig. 4.
Since SNU’s Seoul campus is composed of a total of 208 buildings, building sampling was considered to reduce the total campus size, further minimizing computation requirements while capturing the two-level classification groups. The sample represents the principal specificities of the buildings of interest and is highlighted by the fine red line on the map of Fig. 4. Moreover, this test area was selected according to availability of the observational data (from the SNU ISD database) for gas consumption in 2019, the baseline year; and, comprises five buildings—the gymnasium (building 71, coded 7_B), veterinary medical teaching hospital (building 71-1, coded 3_C), sports education and research building (building 80, coded 2_B), college of veterinary medicine (building 85, coded 2_C), and Woojung Global Social Contribution Center (referred as Woojeongwon, building 153, coded 8_C)—for which no data was missing. The lack of observational data was reflected during the results validation[21].
3.3. Prototype simulation model
Authors chose to employ EnergyPlus, having a better command of the tool, which implied less time spent and the possibility of integrating this software with ENVI-met in future research. Urban designers have used ENVI-met, recognized as one of the most capable tools to predict and simulate microclimates, together with EnergyPlus to include climatic conditions in modeling[29-30].
2D drawing maps were formally requested and acquired from SNU Department of Infrastructure Management and, included information such as the floor outlines, heights, materials for the external and internal walls, floors, rooftops, and insulation and construction years[22]. Table 1. presents detailed 3D model information by class. The outlines of each building were drawn by simplifying the original 2D drawing maps on AutoCAD 2021 and exported to EnergyPlus. The heights of each floor were added and inner rooms were further divided into partitions. Information about outside walls, rooftops, ground floor layers and materials were incorporated to inform of the insulator materials in accordance with Korean construction standards. With the information provided by 2D drawing maps, each room was allocated a type of usage, selected by using ASHRAE standard usage and occupancy templates provided on EnergyPlus. To enable the shading effect, surrounding building blocks and grounds were modeled. This allowed to activate the ground thermal conduction calibration on EnergyPlus by adding objects into 3D models. More detailed information such as the window location and HVAC systems were ignored for model complexity which propelled authors to use default options on EnergyPlus.
3.4. Bayesian calibration method
As aforementioned in the literature review, the Bayesian analysis attempts to estimate the posterior probability distribution for the results of the regressor by assigning a probability prior distribution function to the uncertain values[20]. The Bayesian Theory is captured by the following equation:
(Eq. 1) |
- where:
- - p(θ|y) is the posterior distribution—the conditional probability—also known as the probability of the unknown parameters θ of the model of interest contingent on the observational data y.
- - p(y|θ) is the conditional probability of the model parameters values, known as the likelihood function.
- - p(θ) refers to the prior distribution, the probability of some population parameters.
- - p(y) represents the normalizing factor.
Following this equation, the posterior distribution is computed by multiplying the likelihood function with the prior distribution, divided by the normalizing factor. This product refers to a finalized disposition of knowledge about the model results and allows to compute the relevant means and standard deviations[31-32].
In the present study, all distributions are assumed to be normal ones of the Energy Use Intensity (EUI), defined as the energy use in kilowatt hour (kWh) per square meters of the building area.
The EUIs are outcomes of EnergyPlus simulations, used to estimate the prior distribution of the probability of the EUI inputs, further giving the means of monthly EUIs and standard deviations of the prior distribution as the sample standard deviations of each building’s EUI based on previous observations. The 2019 existing observational data for the buildings gives the estimate of the likelihood function, resulting in the mean likelihood function, the samples’ average value of monthly EUIs. The normalized product of the two distribution functions gives an estimate of the posterior distribution. The expected value, the average of the posterior distribution represents the modified EUIs.
4. Findings
4.1. 3D model simulation results
Fig. 5. illustrates one of the five 3D models built on EnergyPlus for the College of Veterinary. After modeling the archetype building information and surroundings, the simulation engine of the software generated the monthly Energy Use Intensity (EUI), which represents the predictive value of a building, calculated based on the following formula:
(Eq. 2) |
where q is the monthly energy use of a building in kilowatts hour and s is the building area in square meters. After running a total of sixty simulations (twelve months by five buildings) on EnergyPlus, the monthly EUI per building for the year 2019 was acquired. Table 2. gives a summary of the EUIs for each month and each building over the year 2019.
Fig. 6. displays the 2019 monthly energy use intensities (EUIs) of the archetype buildings and the total building complex of the simulation results, prior to the Bayesian calibration and, of the observational data (from the SNU ISD database)[23]. While simulation and observational EUIs for building 71 are close, EUI values for buildings 71-1, 80 and 153 are far from each other, indicating an overestimation of the EUIs resulting from EnergyPlus simulation. On the other hand, the difference in building 85’s EUIs points at an underestimation of the values from the EnergyPlus simulation. Simulation-based EUIs of building 80 and 153 are particularly overestimated during summer.
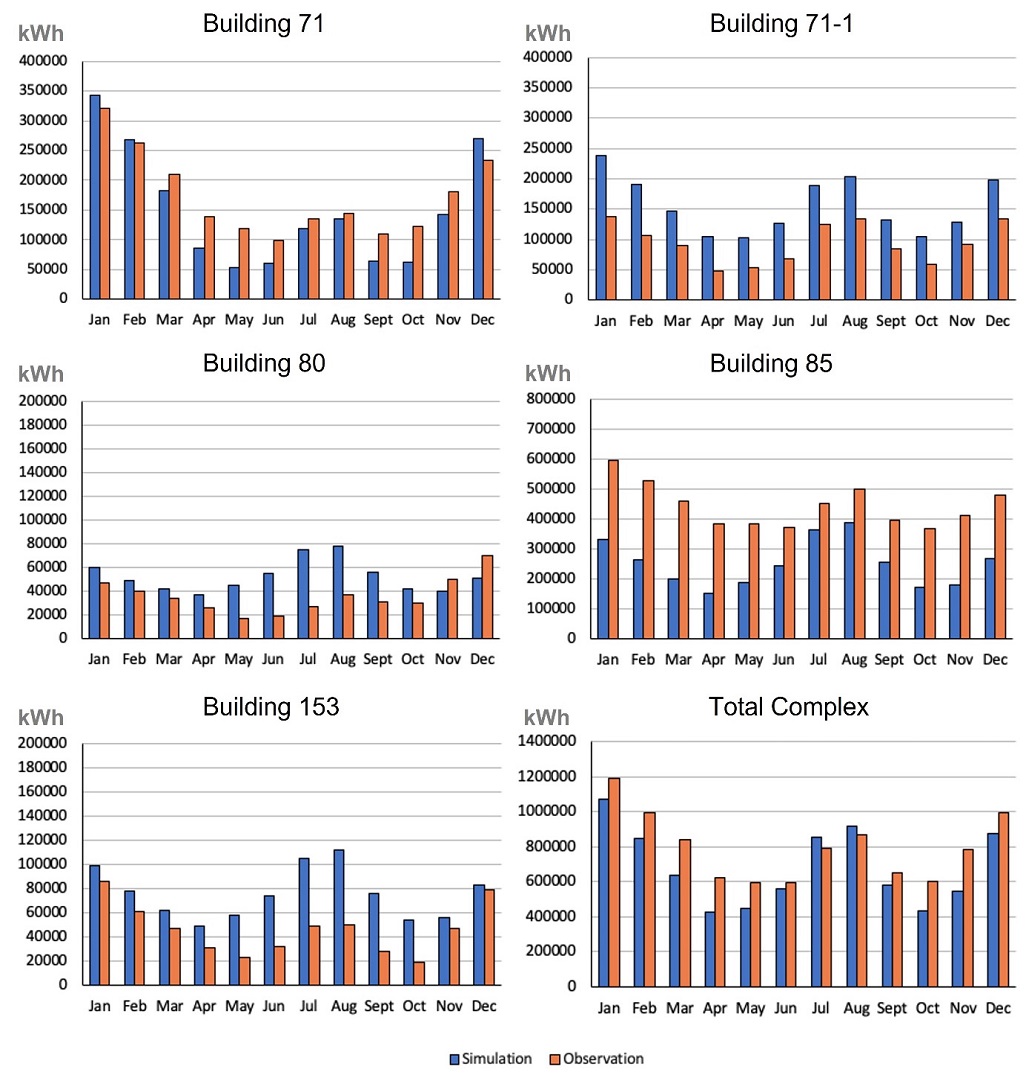
Buildings’ Monthly Energy use (kWh) Comparing Simulation Results and Observational Data for in order Building 71, 71-1, 80, 85, 153 and the Five Buildings Combined
Equipped with the simulation results, the means of monthly EUI prior distributions for each building were computed, leading to a total of 60 prior distributions, the EUIs for twelve months for five buildings. Furthermore, the standard deviations of the monthly energy use intensities of SNU buildings based on the monitored observational data were computed, amounting between 0.5 to 12. Since the average standard deviation had a value of 4, it was selected as the standard deviation of the prior distribution. The samples’ means and standard deviations further gave the likelihood function.
Table 3. further shows the monthly EUIs, under three scenarios—(i) the original simulation results, (ii) the modified simulation results by the Bayesian calibration, (iii) the observational EUI—for each of the buildings of interest. EUIs are modified following the Bayesian calibration executed on Python (ver.3.7). By having information on both the modified EUIs and monitored EUIs of the five buildings, Fig. 7. provides a graphical comparison of the monthly EUIs under these different scenarios. Comparing the different EUIs against each other allows one to determine and measure the effect of the Bayesian calibration, and estimate how close this technique brings the simulation-based EUIs to the actual EUIs. Except for buildings 80 and 153, the Bayesian calibration brought the simulation-based EUIs closer to the observational EUIs.

2019 Monthly Energy Use Intensity per Archetype Building of Interest by Scenario; Simulation, Modified, Observation (kWh/㎡)
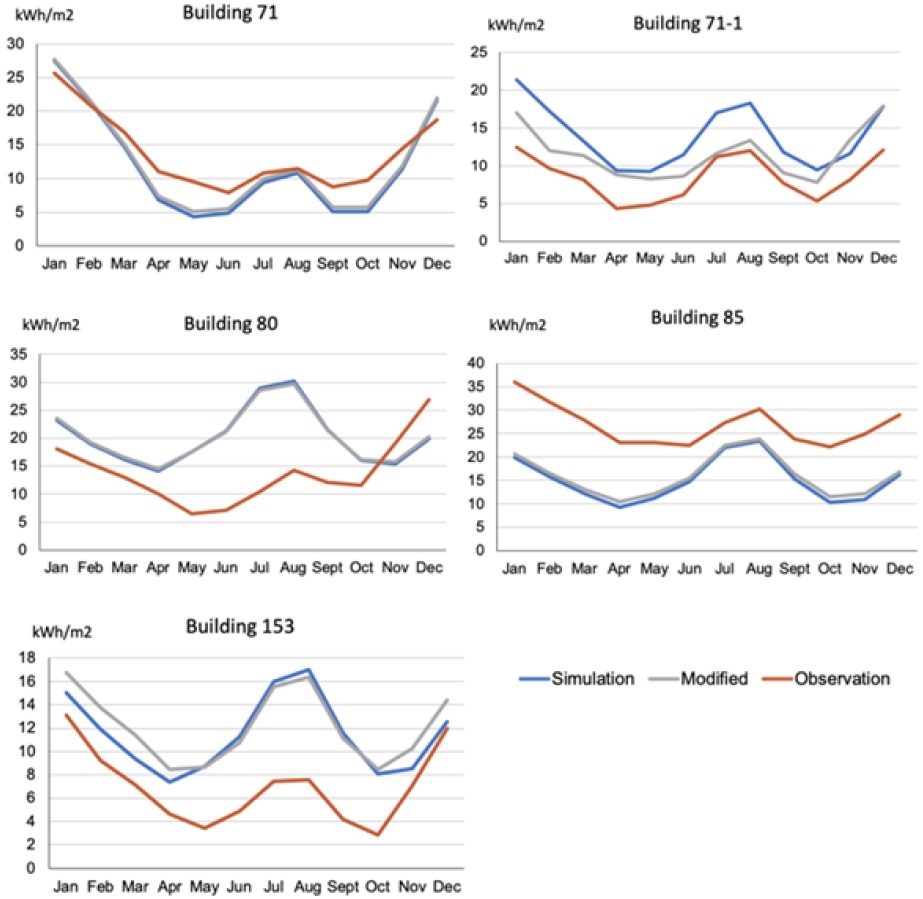
Comparison of EUIs (Original, Modified and Observational) for Buildings 71, 71-1, 80, 85 and 153Note: The above graphs show the simulation results compared with the observational data for, in order, the buildings 71, 71-1, 80, 85, 153, for the year 2019 from January to December in hour kilowatts per cubic meter.
4.2. Results validation
In order to validate the findings, the coefficient of variance of root mean squared error (CVRMSE)—mean-based normalized RMSE which is ‘the sample standard deviation of the differences between [the] modelled and baseline values’ and represents an estimate of accuracy of the calibrated values—was employed. This measure is not only recommended in ASHRAE’s guideline but has been widely used as a validation method of energy models in the literature[8]. The CVRMSE equation is denoted as follow:
(Eq. 3) |
The CVRMSE of the original simulation results amounted to 62.1%, and 56.5% for the EUIs calibrated with the Bayesian technique, providing evidence for a decrease in instability in the relationship between the EUIs which is attributed to the Bayesian calibration. Nevertheless, ASHRAE guideline 14 recommends a CVRMSE of 15% as an appropriate percentage to determine monthly energy consumption prediction, which represents a much smaller value in comparison to this study’s CVRMSE estimates.
5. Discussion
This study has thrown light on the role of Bayesian calibration in addressing uncertainty in bottom-up UBEM, particularly archetype modeling, by showing that integrating Bayesian statistics with model the observational data and enhancing results accuracy. Nevertheless, against previous literature findings, the effect of the Bayesian calibration was not as large as expected, where the simulation results’ CVRMSE, while improved post-calibration, is yet far from ASHRAE’s recommended value by more than two-fold. These findings offer enough support to reject the null hypothesis and authors can claim that the Bayesian calibration does not represent a sufficient and adequate method to resolve uncertainties and improve accuracy in this case study.
Uncertainty issues arise from different sources such as the incorrect input of parameters and misspecification of building system materials and parameters. In this research, uncertainty arises from the absence of input of certain parameters, and the lack of specification for some system parameters, due to the essence of archetype modeling, deprivation of some data, and software’s limitations in integrating microclimatic conditions. For instance, information of heating, ventilation, and air conditioning (HVAC) systems for the five buildings was unavailable, which propelled researchers to use HVAC systems default settings on EnergyPlus. Employing default settings is likely to have affected heating, cooling and hot water energy consumption. During summer, simulation outputs indicated an overestimation of energy use intensity which revealed the potential influence of using default settings for HVAC systems.
Urban designers are prompted to integrate urban climatic parameters into modeling to endorse sustainable urban planning actions[33]. In this study’s 3D design process, terrain and surrounding buildings were added to the 3D models to take into consideration the geographic contexts of each archetype building. However, microclimatic parameters were not included because of EnergyPlus drawbacks in adding such layers. SNU campus is located at the northwest of the Gwanaksan mountain which implies the existence of peculiar climatic characteristics. Integrating EnergyPlus and ENVI-met was considered to model microclimatic conditions, but dropped due to time-constraints which leaves room for further modeling improvement.
In addition, the standard deviations of the archetype samples impact the levels of modification of the modified EUIs; the smaller the sample deviation based on the archetype sample, the larger the EUIs modifications caused by the Bayesian calibration. The standard deviation, which measures the spread of the data distribution, is affected by outliers in the distribution and affects the standard error. Thus, archetype characterization represents a fundamental component of archetype modeling in which buildings under the same archetype category shares the same important characteristics that influence energy consumption. A sophisticated and well-defined archetype characterization results in smaller standard deviation among the sample distribution by reducing the risk of having outliers and hence, increases the effectiveness of the Bayesian calibration. In this study, building 153 represented an outlier in the 8_C archetype sample distribution, where the observational EUI and modified EUI had large, measurable discrepancy.
6. Conclusion
In consideration of the gap in research on uncertainties in urban building energy modeling, this paper attempted to address accuracy issues by using the Bayesian calibration method, as recommended in a few research papers, in order to modify and improve the accuracy of the monthly energy use intensities (EUIs) of five archetype buildings of Seoul National University Campus for 2019. The buildings were first modeled on EnergyPlus which permitted to calculate the monthly EUIs values, which were further exported and calibrated by the Bayesian method on Python. Against the preliminary assumptions, the Bayesian calibration method only slightly improved the simulation values, by reducing a small gap between the simulation results and the observational data. Thus, this paper pointed out the shortcoming of archetype modeling when this one did not include some parameters, particularly, characteristics of HVAC systems, and highlighted the need for better archetype characterization, and validation when testing models. Without any sort of validation, uncertainties and errors go unnoticed and cannot be quantitatively verified.
The Bayesian calibration may be considered to address uncertainties; however, it is not a one-size-fits- all solution to uncertainties caused by to the lack of input of some parameters which are crucial to the archetype characterization. In this case study, the suggested Bayesian method does not represent a complete and adequate calibration tool as authors cannot confidently claim its accuracy.
Further research should strongly focus efforts on archetype characterization and carefully consider which parameters must be included in archetype modeling in order to estimate energy consumption. HVAC systems should be parameterized in building modeling and cannot be reduced to default settings as this process would distort simulation results regardless of the further use of calibration methods. Future research could benefit from incorporating a sensitivity analysis via the Morris Method, with the objective to determine which variables influence the most the simulation findings and accordingly, tailor the Bayesian calibration method in a more suited way. Calama-González et al. (2021) assessed indoor thermal comfort employing training periods of hourly temperature data which were calibrated by the Bayesian method, following a sensitivity analysis. The Bayesian calibration was attributed an 80 percent improvement for the CVRMSE when paired with the Morris Method, in contrast to a 40% enhancement in accuracy without the Morris Method in other studies[21].
By consequence, authors conclude that the Bayesian calibration method may improve accuracy in studies of building energy modeling and address uncertainties issues. Nevertheless, the Bayesian method remains limited, as showed in this paper’s case study, when authors fail to identify which variables influence the most building energy consumption, which could be further improved by using a sensitivity analyses in future research.
References
-
C. F. Reinhart, C. C. Davila, Urban building energy modeling–A review of a nascent field, Building and Environment, 97, 2016, pp.196-202.
[https://doi.org/10.1016/j.buildenv.2015.12.001]
-
M. Brøgger, P. Bacher, K. B. Wittchen, A hybrid modelling method for improving estimates of the average energy-saving potential of a building stock, Energy & Buildings, 199, 2019, pp.287-296.
[https://doi.org/10.1016/j.enbuild.2019.06.054]
-
L. G. Swan, V. I. Ugursal, Modeling of end-use energy consumption in the residential sector: A review of modeling techniques, Renewable and Sustainable Energy Reviews, 13, 2009, pp.1819-1835.
[https://doi.org/10.1016/j.rser.2008.09.033]
-
G. Calleja Rodríguez, A. Carrillo Andrés, F. Domínguez Muñoz, J. M. Cejudo López, Y. Zhang, Uncertainties and sensitivity analysis in building energy simulation using macroparameters, Energy and Buildings, 67, 2013, pp.79-87.
[https://doi.org/10.1016/j.enbuild.2013.08.009]
-
M. Gholami, D. Torreggiani, P. Tassinari, A. Barbaresi, Narrowing uncertainties in forecasting urban building energy demand through an optimal archetyping method, Renewable and Sustainable Energy Reviews, 148, 2021, p.111312.
[https://doi.org/10.1016/j.rser.2021.111312]
- T. A. Reddy, Literature Review on Calibration of Building Energy Simulation Programs: Uses, Problems, Procedures, Uncertainty, and Tools, ASHRAE Transactions, 112, 2006, pp.226-240.
-
Y. Sun, Y. Heo, M. Tan, H. Xie, C. F. J. Wu, G. Augenbroe, Uncertainty quantification of microclimate variables in building energy models, Journal of Building Performance Simulation, 7(1), 2014, pp.17-32.
[https://doi.org/10.1080/19401493.2012.757368]
-
D. Chakraborty, H. Elzarka, Performance testing of energy models: are we using the right statistical metrics, Journal of Building Performance Simulation, 11(4), 2018, pp.433-448.
[https://doi.org/10.1080/19401493.2017.1387607]
-
F. Johari, G. Peronato, P. Sadeghian, X. Zhao, J. Widén, Urban building energy modeling: State of the art and future prospects, Renewable and Sustainable Energy Reviews, 128, 2020, p.109902.
[https://doi.org/10.1016/j.rser.2020.109902]
-
M. Ferrando, F. Causone, T. Hong, Y. Chen, Urban building energy modeling (UBEM) tools: A state-of-the-art review of bottom-up physics-based approaches, Sustainable Cities and Society, 62, 2020, p.102408.
[https://doi.org/10.1016/j.scs.2020.102408]
-
N. Ghiassi, A. Mahdavi, Reductive bottom-up urban energy computing supported by multivariate cluster analysis, Energy and Buildings, 144, 2017, pp.372-386.
[https://doi.org/10.1016/j.enbuild.2017.03.004]
-
S. Risch, P. Remmen, D. Müller, Influence of data acquisition on the Bayesian calibration of urban building energy models, Energy & Buildings, 230, 2021, p.110512.
[https://doi.org/10.1016/j.enbuild.2020.110512]
-
T. Zhao, J. Xu, C. Zhang, P. Wang, A monitoring data based bottom-up modeling method and its application for energy consumption prediction of campus building, Journal of Building Engineering, 35, 2021, p.101962.
[https://doi.org/10.1016/j.jobe.2020.101962]
-
J. Sokol, C. Cerezo Davila, C. Reinhart, Validation of a Bayesian-based method for defining residential archetypes in urban building energy models, Energy and Buildings, 134, 2017, pp.11-24.
[https://doi.org/10.1016/j.enbuild.2016.10.050]
-
W. L. Oberkampf, S. M. DeLand, B. M. Rutherford, K. V. Diegert, K. F. Alvin, Error and uncertainty in modeling and simulation, Reliability Engineering and System Safety, 75, 2002, pp.333-357.
[https://doi.org/10.1016/S0951-8320(01)00120-X]
-
W. Tian, Y. Heo, P. de Wilde, Z. Li, D. Yan, C. Park, X. Feng, G. Augenbroe, A review of uncertainty analysis in building energy assessment, Renewable and Sustainable Energy Reviews, 93, 2018, pp.285-301.
[https://doi.org/10.1016/j.rser.2018.05.029]
-
A. Chonga, K. Menberg, Guidelines for the Bayesian calibration of building energy models, Energy & Buildings, 174, 2018, pp.527-547.
[https://doi.org/10.1016/j.enbuild.2018.06.028]
-
H. Lim, Z. Zhai, Influences of energy data on Bayesian calibration of building energy model, Applied Energy, 231, 2018, pp.686- 698.
[https://doi.org/10.1016/j.apenergy.2018.09.156]
-
C. Zhua, T. Wei, B. Yinc, Z. Lia, J. Shia, Uncertainty calibration of building energy models by combining approximate Bayesian computation and machine learning algorithms, Applied Energy, 268, 2020, p.115025.
[https://doi.org/10.1016/j.apenergy.2020.115025]
-
W. M. Bolstad, Understanding computational Bayesian statistics, Wiley, 2010.
[https://doi.org/10.1002/9780470567371]
-
C. M. Calama-González, P. Symonds, G. Petrou, R. Suárez, A. L. Léon-Rodríguez, Bayesian calibration of building energy models for uncertainty analysis through test cells monitoring, Applied Energy, 282, 2021, p.116118.
[https://doi.org/10.1016/j.apenergy.2020.116118]
- Seoul National University Infrastructure Management Department, 2D drawings of buildings 71, 71-1, 80, 85, and 153, 2021.
-
서울대학교 지속가능발전연구소, Greenhouse Gas & Energy, https://isd.snu.ac.kr/isd/eng_ISD2.php, , 2021.11.08.
SNU Institute for Sustainable Development, Greenhouse Gas & Energy, https://isd.snu.ac.kr/isd/eng_ISD2.php, , 2021.11.08. -
U. Ali, M. Haris Shamsi, C. Hoare, E. Mangina, J. O’Donnell, A data-driven approach for multi-scale building archetypes development, Energy & Buildings, 202, 2019, p.109364.
[https://doi.org/10.1016/j.enbuild.2019.109364]
-
M. Heine Kristensen, R. Elbæk Hedegaard, S. Petersen, Hierarchical calibration of archetypes for urban building energy modeling, Energy & Buildings, 175, 2018, pp.219-234.
[https://doi.org/10.1016/j.enbuild.2018.07.030]
-
T. Alves, L. Machado, R. Goncalves de Souza, P. de Wilde, A methodology for estim ating office building energy use baselines by means of land use legislation and reference buildings, Energy and Buildings, 143, 2017, pp.100-113.
[https://doi.org/10.1016/j.enbuild.2017.03.017]
- J. H. Yoon, B. H. Lee, M. S. Yeo, K. W. Kim, Classification of Energy Consumption Patterns in University Buildings Using Change Point Model and Analysis According to Energy Impact Factors, Journal of the Architectural institute of Korea structure & construction, 33(11), 2017, pp.77–78.
-
국가법령정보센터, https://law.go.kr, , 2021.11.08.
National Law Information Center, https://law.go.kr, , 2021.11.08. -
J. Balter, N. Alchapar, E. Correa, C. Ganem, Modelado urbano microclimático para la simulación termo-energética de viviendas en edificios en altura en Mendoza, Argentina. Integración del software ENVI-met y EnergyPlus, Informes de la Construcción, 73, 2021, p.561.
[https://doi.org/10.3989/ic.76749]
-
D. B. Crawley et al., EnergyPlus: creating a new-generation building energy simulation program, Energy and Buildings, 33(4), 2001, pp.319-331.
[https://doi.org/10.1016/S0378-7788(00)00114-6]
-
M. Manfren, N. Aste, R. Moshksar, Calibration and uncertainty analysis for computer models – A meta-model based approach for integrated building energy simulation, Applied Energy, 103, 2013, pp.627-641.
[https://doi.org/10.1016/j.apenergy.2012.10.031]
-
R. van de Schoot et al., Bayesian statistics and modelling, Nature Reviews: Methods Primers, 1(1), 2021, pp.1-26.
[https://doi.org/10.1038/s43586-020-00001-2]
-
N. H. Wong, S. Kardinal Jusuf, C. L. Tan, Integrated urban microclimate assessment method as a sustainable urban development and urban design tool, Landscape and Urban Planning, 100, 2011, pp.386-389
[https://doi.org/10.1016/j.landurbplan.2011.02.012]